Target Function: Time Window and Operations on Events
The imput transformations allowed in functions
Check This First!
This article refers to BaseModel accessed via Docker container. Please refer to Snowflake Native App section if you are using BaseModel as SF GUI application.
In this article, we will cover the transformations which we can apply to events and entity attributes in order to obtain the output type and value suitable for your business scenario.
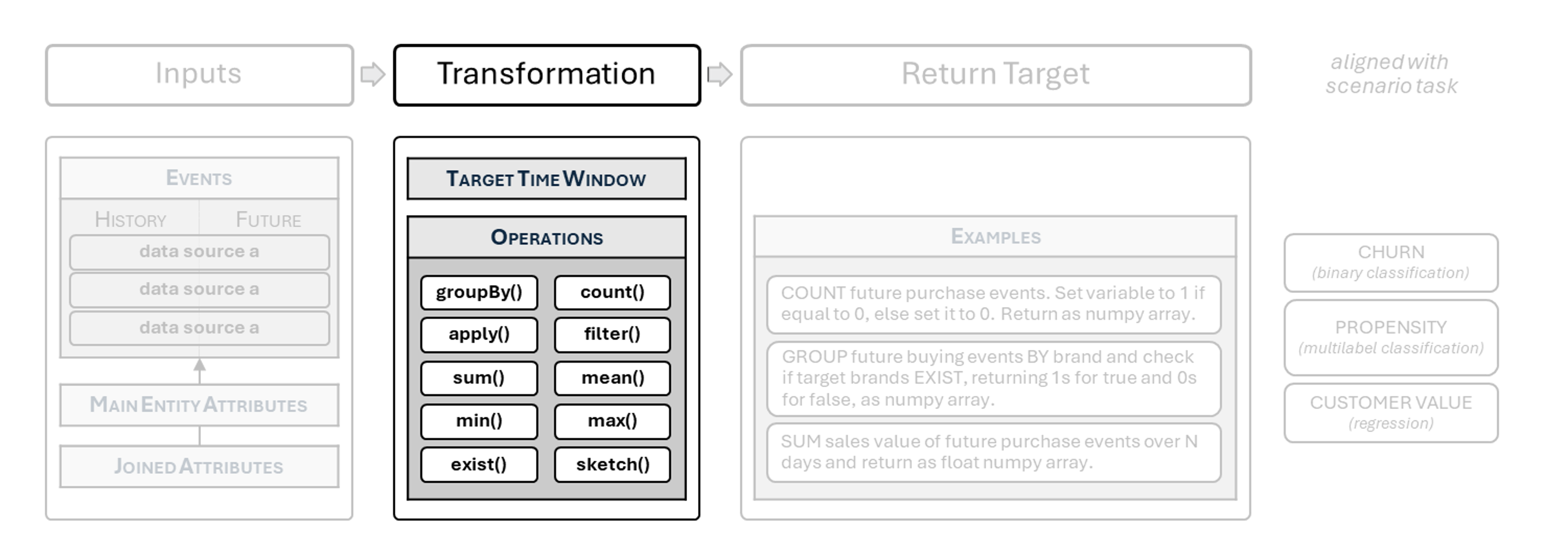
Target Time Window
The foundation model treats all events after the temporal split as "future" and trains to predict them. However, when building scenario models, we typically want the target function to focus on a particular period in the future
, such as:
- Which customers will lapse, i.e., fail to make an interaction over a number of days.
- How much a customer will spend over a period of time.
This means that for most scenario models, we need to constrain the future to a time window of desired length immediately following the temporal split for a given entity. It is also recommended to return None for those entities where the random split point leaves too short a window to train the model with the appropriate target.
The example below demonstrates this in practice:
def target_fn(_history: Events, future: Events, _entity: Attributes, _ctx: Dict) -> np.ndarray:
target_window_days = 21
if has_incomplete_training_window(_ctx, target_window_days):
return None
future = next_n_days(future, _ctx[SPLIT_TIMESTAMP], target_window_days)
Exception
Scenario models for recommendation tasks do not predict events over a time window. Instead, they aim to predict the events most likely to happen in the immediate future irrespective of time (e.g., "the next basket").
Trimming the future to a time window should not be performed for recommendation tasks!
Operations on events
The following operations can be implemented within the target function directly on event objects, both history
and future
:
-
count()
Calculates the number of events.
Returns:Int
, count of eventsExample:
churn = 0 if future['product_buy'].count() > 0 else 1
-
sum(column: str)
Sums values for specified column.
Argument: a column which values will be aggregated into sum.
Returns: float: sum of the values in the specified column.Example:
future['transactions'].sum(column='purchase_value')
-
mean(column: str)
Calculates mean value for specified column.
Argument: a column which values will be aggregated into mean.
Returns: float: mean of the values in the specified column.Example:
future['transactions'].mean(column='price')
-
min(column: str)
Finds a min value in a specified column.
Argument: a column for which min value will be extracted.
Returns: float: min value in the specified column.Example:
future['transactions'].min(column='purchase_value')
-
max(column: str)
Finds a max value in a specified column.
Argument: a column for which max value will be extracted.
Returns: float: max value in the specified column.Example:
future['transactions'].max(column='purchase_value')
-
apply(func: Callable[[Any], Any], target: str)
Applies functionfunc
to atarget
column.
Returns:DataSourceEvents
, events with columntarget
transformed by thefunc
.Example:
future['product_buy'].apply(lambda x: x.lower()), target='brand')
-
filter(by: str, condition: Callable[[Any], bool])
Filters events based on thecondition
checked against columnby
.
Returns:DataSourceEvents
, events filtered based on thecondition
checked against columnby
.Example:
future['transactions'].filter(by="PROD_ID", condition=lambda x: x in products_in_campaign)
-
groupBy(by: str | list[str])
Groups the events by values in a column (or list of columns) provided afterby
.
Returns:EventsGroupBy
: a proxy object.Note
groupBy
requires one of the operators listed in the next section to return anything.Example:
future['product_buy'].groupBy('brand').exists(groups=['Nike', 'Adidas'])
Operations on grouped events
You can also do the following operations applying them to grouped events (EventsGroupBy
). This is useful when you want to e.g. check for existence of purchases from categories or brands etc.:
-
count(normalize: Optional[bool] = False, groups: Optional[List[Any]] = None)
Counts elements in each group.
Arguments:- normalize : scales counts so that they sum to 1 (boolean, default: False)
- groups : limit grouping to the list provided (a list, default: None)
Returns:
Tuple[np.ndarray, List[str]]
, a tuple with count of elements per each group and group names.Example:
future['purchases'].groupBy('brand').count(normalize=True, groups=['Garmin', 'Suunto'])
-
sum(target: str, groups: Optional[List[Any]] = None)
Sums the values of the columntarget
in each group.
Arguments:- target : a column to apply the grouping operation to (str, required)
- groups : limit grouping to the list provided (a list, default: None)
Returns:
Tuple[np.ndarray, List[str]]
, a tuple with sum of elements per each group and group names.Example:
future['transactions'].groupBy('category').sum(target='purchase_value')
-
mean(target: str, groups: Optional[List[Any]] = None)
Computes the mean of the values of the columntarget
in each group.
Arguments:- target : a column to apply the grouping operation to (str, required)
- groups : limit grouping to the list provided (a list, default: None)
Returns:
Tuple[np.ndarray, List[str]]
, a tuple with mean of elements per each group and group names.Example:
future['transactions'].groupBy('brand').mean(target='purchase_value')
-
min(target: str, groups: Optional[List[Any]] = None)
Computes the minimum of the values of the columntarget
in each group.
Arguments:- target : a column to apply the grouping operation to (str, required)
- groups : limit grouping to the list provided (a list, default: None)
Returns:
Tuple[np.ndarray, List[str]]
, a tuple with min value of elements per each group and group names.
Example:future['transactions'].groupBy('category').min(target='price')
-
max(target: str, groups: Optional[List[Any]] = None)
Computes the maximum of the values of the columntarget
in each group.
Arguments:- target : a column to apply the grouping operation to (str, required)
- groups : limit grouping to the list provided (a list, default: None)
Returns:
Tuple[np.ndarray, List[str]]
, a tuple with max value of elements per each group and group names.Example:
future['transactions'].groupBy('store').max(target='value')
-
exists(self, groups: List[Any])
Checks if any of thegroups
is empty.
Arguments:- groups : limit grouping to the list provided (a list)
Returns:
Tuple[np.ndarray, List[str]]
, a tuple with array indicating existence of the elements per each group and group names.Example:
future['transactions'].groupBy('brand').exists(groups=TARGET_BRANDS)
-
apply(self, func: Callable[[np.ndarray], Any], default_value: Any, target: str, groups: Optional[List[Any]] = None)
Applies a functionfunc
to each group.
Arguments:-
func (Callable[[np.ndarray], Any]): Function to apply.
-
default_value (Any): Default output value.
-
target (str): Column to apply the grouping operation to.
-
groups : limit grouping to the list provided (a list)
Returns: Tuple [Any, List[str]], a tuple with values returned by
func
per each group and group names.
Example:future['product_buy'].apply(lambda x: x.lower()), target='brand')
-
Updated 6 days ago